Think about the maps you use on your phone. They provide useful, contextual information. You can get directions, see the roads, check satellite and street imagery, search for businesses, find traffic cameras, check congestion and more. This data is valuable. Accenture says OpenStreetMap (which provides data to most mapping services) is worth $1.67 billion.
Autonomous vehicles need this information with context to make good decisions in response to what is happening around them in real time. For example, they must be able to identify difficult-to-see elements, changing road width or road surfaces. AV must also make good decisions regarding other road users and predict and react to unexpected events, such as a child on the road.
Only some of this mapping information can be provided by public data stacks and Waze-like crowdsourced information. This is why effective sensor technologies and artificial intelligence (AI) are essential to comprehend ambient events.
“The pathway to increased vehicle autonomy will be largely built on gradual feature and capability advancements,” says Matt Arcaro, IDC Research Manager, Next-Generation Automotive and Transportation Strategies.
What approaches exist?
While Tesla relies on video-based systems, most autonomous vehicle makers employ both Lidar and video capture to acquire the data they need. The former for its location accuracy, the latter to add depth perception. Lidar is accurate within centimeters, component costs are plummeting, and it can create 3D maps for vehicles. The sensor range of Lidar is around 200m, though it cannot detect the velocity of an object. Lidar doesn’t require ambient light and is less susceptible to rain or fog through its use of near infrared signals. Apple, Tesla, Ford, Volkswagen, Microsoft, Hyundai and others are investing heavily in Lidar, transforming Lidar research into an arms race as most explore it.
China’s official autonomous driving test report revealed that in 2020, there were 2.2 million kilometers of road test, up 113% since 2019. It also showed 71% of vehicles tested in closed training grounds used Lidar sensors. Supplemental technologies include thermal imaging and 4D imaging radar within car mapping and AV systems. The report confirms the most common causes of disengagement (when the AI fails and a human test driver takes charge) were vehicle congestion, illegal lane changes, illegal parking and unpredictable pedestrian activity.
Improving Lidar driver assistance
Intel is working closely with Mobileye to develop a Lidar system-on-chip (SoC) for autonomous vehicles starting in 2025. Mobileye has a software-defined, automated imaging radar technology called Road Experience Management (REM). The company claims it has mapped nearly 1 billion kilometers globally at a rate of 8 million kilometers daily and hopes that the system will become standardized. To achieve this, the company is leveraging the driver assistance solutions it already brings to market inside nearly a million vehicles from partners including BMW, Nissan and Volkswagen.
Unlike Tesla, which captures vast amounts of data for analysis in the cloud, Mobileye’s system gathers data, such as road geometry and what nearby vehicles are doing, and processes it in the onboard system. It shares compact summaries (10kb per kilometer) with the cloud for analysis to deliver detailed insights, such as the location of curbs, street signs or even how actual driving patterns differ from the road markings. Mobileye showed REM’s benefits in 2020 when it used it to train autonomous vehicles to start driving in Munich and Detroit after just a few days’ safety training.
Challenging AV driving conditions
Even with technologies like these, building the mountain of inherent core data to the degree of accuracy that AV requires is a huge task given so many local variables. In part, that’s why Toyota says truly autonomous vehicles will require 500 billion miles of driving data, which will take 20 years to gather.
The need to gather so much data is prompting moves to share data. AV developers know they will not be forgiven if manufacturer-led refusal to share information causes injury. In Germany, the federal government plans a shared mobility data space, providing useful information, such as weather, traffic or even roadworks, to inform future transportation systems.
There are computational challenges to handling all this information. The AI in the autonomous vehicle must be capable of processing multiple gigabytes of raw data in near real time and must make the right judgment calls based on the information it finds, which means vehicles will require cutting-edge processors.
However, the need for processors poses unexpected problems. Global processor demand is so high that some of the world’s biggest car manufacturers this year cut vehicle production in response to silicon shortages. Component supply will slow production, which means AV and non-AV vehicles must learn to safely share the road, making the capacity to make the right on-road decisions using maps, sensors and algorithms even more critical.
Fragmentation may also become a problem. As manufacturers develop their own AV technologies, they must ensure these are compatible with others, given that the road is a shared resource. Inevitably, vehicle-to-vehicle communication will be part of this, which is why manufacturers such as Toyota, Mazda and PSA Group are working with mobile networks, including Orange.
Last-mile transit
While developing solutions to AV’s complex challenges, the industry is focusing on what it hopes are relatively low-risk ways to introduce first-generation systems. McKinsey & Co. claims that around half of all passenger miles are journeys just one to five miles long. Many of these are in low-traffic environments ideal for autonomous shuttle vehicles, which you’ll now find in universities, industrial parks and airports.
Mobileye’s plans include the use of LiDAR in driverless taxis, while Volkswagen intends to launch a ride-hailing service based on AV models of its iconic camper vans in 2025. These systems will likely contribute valuable contextual and real-world road usage/mapping data to inform second-generation, truly autonomous systems when they appear.
Orange is helping the leading developers and manufacturers in automotive to develop autonomous vehicles. Learn more about our partnerships with Mobileye, Toyota and PSA.
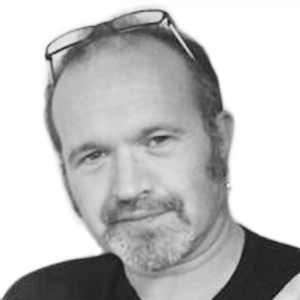
Jon Evans is a highly experienced technology journalist and editor. He has been writing for a living since 1994. These days you might read his daily regular Computerworld AppleHolic and opinion columns. Jon is also technology editor for men's interest magazine, Calibre Quarterly, and news editor for MacFormat magazine, which is the biggest UK Mac title. He's really interested in the impact of technology on the creative spark at the heart of the human experience. In 2010 he won an American Society of Business Publication Editors (Azbee) Award for his work at Computerworld.