Predictive maintenance has the ability to improve product quality, increase productivity, reduce downtime and costs while speeding up decision making - but despite all these benefits there is still more debate than action when it comes to actually deploying solutions in factories today.
Why? Because every factory is different and predictive maintenance has to be customized accordingly and must fit individual process frameworks. This makes the adoption in many cases easier said than done. In addition, manufacturing plants’ IT infrastructures aren’t necessarily geared up to running predictive maintenance applications. Many older machines are not equipped with the required sensors and have not been connected yet. Often these machines are a decade old and the operating systems even older. The vast majority of data has yet to move to the cloud, at least to build up initial models. This is primarily down to worries around security, especially at the Program Logical Controller level which is responsible for controlling production lines, for example. In addition there seems to be a constant shortage of data scientists and domain experts
On the other side, unintended production stoppages costs manufacturing plants potentially billions of dollars in lost revenue. The most recent in-depth report on manufacturing downtime carried out by Neilsen Research back in 2006 found that stoppage in car manufacturing plants can cost up to $1.3 million per hour. And a 2017 survey of UK manufacturers by field service management company Oneserve found that 3 per cent of all working days are lost annually due to faulty machinery, equating to 49 hours of work.
Spare part inventories can also be a juggling act for in-house maintenance teams who don’t want to tie up working capital in spare parts. This makes predictive maintenance a virtually indispensable application for manufacturing. But there are still awareness issues, which have also hobbled its progress. Manufacturing plants are often conservative in their outlook, accustomed to manual maintenance on the ground. Infrastructures and resources also vary from country to country when it comes to implementing a predictive maintenance strategy.
Maintenance and reliability
Predictive maintenance adds value to manufacturing businesses by predicting events that management may not have envisaged. At the same time, it maximizes the life of parts and machines, while avoiding unplanned stoppages and reducing planned downtime as far as possible.
But there are hurdles to overcome. Manufacturing plants run on two key criteria: that they are safe and they are constantly producing goods, with only limited windows for maintenance . If this is happening they see no need for rapid change. Components forming part of the production line are certified and difficult to change and the operating technology isn’t attached to anything in the outside world. It has a one-way gateway to communicate out and limited communications in. Predictive maintenance applications need cloud, however, where everything is online and constantly connected.
Anticipating maintenance activities
In addition there is the frequently asked question: “Is this really working and paying off?” It might be a good idea to look to some services scenarios, which are already up and running and show the impact of predictive maintenance.
Orange Business’ customer, French e.l.m. leblanc, a gas boiler and water heater manufacturer and subsidiary of the Bosch Group, has introduced predictive maintenance to its product line, for example. The main target here is obviously more on providing additional services and to gain insight for R&D. But the base scenario, is not so different from the requirements we see also on the factory floor.
Orange has provided e.l.m. leblanc with a customized IoT platform on Microsoft Azure which delivers a tailored solution for remote monitoring, alongside curative and predictive maintenance. The remote monitoring solution collects the boiler’s data and alerts technicians if any problems arise. This enables efficient maintenance intervention, with fine-tuned predictions on the probable causes of failure based on real-time data analytics. Pre-emptive alerts can also be raised by the platform through predictive maintenance algorithms. Benefits include optimization of intervention processes, and increased end-customer satisfaction, thanks to innovative and responsive customer support.
e.l.m. leblanc’s case study shows that predictive maintenance is very much here as a technology. It is now up to manufacturers to recognize the value of reliability optimization, and we are aware that this will take time, with major adoption unlikely for the next three years. With predictive maintenance being adopt for selected and most critical components first.
Looking ahead
It is important to point out that predictive maintenance isn’t a silver bullet to solve all manufacturing’s production issues. It will not change the flexibility of what and how something is produced. Neither will technology alone provide you with a successful maintenance strategy. Change management also needs to address processes and internal changes, including how they will affect workers.
Manufacturing plants that plan their predictive maintenance journey will see a reduced downtime, a cut in unnecessary maintenance costs and reduce operational risks, which all have a major impact on the bottom line.
Find out how to Increase speed and agility at every phase of the manufacturing lifecycle to achieve operational excellence and improve competitive advantage here
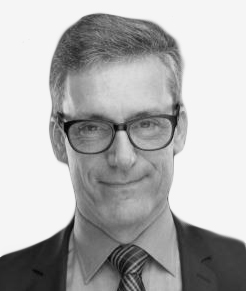
Werner joined Orange Business in 2017 as Head of the IoT Industrial Vertical, to support the development and deployment of the Orange verticalized business development strategy.
During his more than 20 years' experience, he has developed extensive experience in B2B across Europe, with a focus on Industry 4.0 and digitization. Prior to joining Orange Business, Werner spent more than fifteen years with Microsoft in Germany, gaining strong IoT expertise. He has a solid marketing and business development background, complemented by great management experience.