There’s a meme that often pops up on social media, particularly those aimed at professional networking and career development. It shows a slide with the message that businesses are not business to consumer (B2C) or business to business (B2B), they’re human to human.
Pre-pandemic, it might have seemed like a bit of a gimmick. Now, it appears increasingly true. The days of businesses only thinking about who they were selling to directly are long gone. Nowadays, it doesn’t matter what type of business you are, whether a retailer, a wholesaler, a distributor or a manufacturer: you’re expected to know everything about the end customer. Why? Because as expectations of a good customer experience have increased, so every part of the value chain has had to adapt to fulfill these new demands.
Then there’s those historically B2B businesses that are moving rapidly to a direct-to-consumer (D2C) model. They, too, have challenges in this brave new world; they need to implement a new way of reaching consumers, without upsetting their traditional retail sales channels.
It doesn’t matter if you’re a traditionally B2B business that’s being told it needs to be more consumer-focused, or one trying out D2C for the first time, you need to know the end customer. It is expected: one study found that 80% of consumers want personalization from retailers, and that tailoring will only come from knowing the customer. How do you do that? With data.
Know your customer in detail
The data opportunity for businesses, irrespective of customer, is immense. Ultimately, we have to ask ourselves, why do people buy from one organization over another? Because the chosen seller makes life easier. That might be because the price is cheaper; it might be because the buying process was simpler than that of competitors, or because the offer was tailored to the buyer. Whatever the reason, those businesses that are seen to help customers are the ones that will win.
To do this, they need to know what buyers want. How do they find that out? By gathering data from different sources (which can include customer feedback surveys, market research, social media, sentiment analysis, interactions, past buying behavior, segmentation, their own channels including contact centers, connected devices, partners and so on), analyzing it and applying those insights.
Some brands, particularly retailers and banks dealing directly with consumers, have a head start. They have years of data gathering and analysis to draw upon.
But this doesn’t necessarily matter. The true value is being able to use data efficiently to act in real time. That starts by taking the data you have, learning from it and putting it to use to create better experiences. It’s about centralizing the data you have to improve understanding of the customer journey – for example, when it comes to communications, whatever messages the customer receives need to be relevant to them, worth the effort to the business (i.e., the product or service in question needs to have the right value) and involve the right suppliers or partners where necessary. It’s a constant juggling act and one all businesses need to master.
The fallibility of data
But there’s another factor that businesses targeting new audiences need to consider – the unpredictability of people. For instance, past buying behavior might tag a customer as medium value, someone who is never going to buy at a premium level or past a certain price point. But what if this person is then found browsing exactly those products?
Looking purely at past data, this behavior doesn’t make much sense, and so an outcome becomes harder to predict.
Bringing psychology and real-time intelligence together
This is where an understanding of buyer psychology needs to be combined with artificial intelligence and machine learning. It’s about introducing the notion of serendipity, where a product, service or experience is unexpected and appeared to involve chance. Research by the Universities of Sydney and Florida and Rutgers University found that the feelings generated from these encounters are positive. Many people want to find and try new things that don’t necessarily directly relate to what they’ve previously viewed or bought.
More businesses are starting to incorporate them as well – Netflix has introduced its "Surprise Me" button, while there are more and more product-subscription services, covering everything from t-shirts and make up to books, appearing every day.
These supposedly random choices are actually based on existing tastes and choices. Companies that introduce AI and ML tools, fed with relevant data, can start to predict when customers want to see something new, or if they are looking at something they previously haven’t, how likely they are to buy.
For our customer browsing premium products, a company combining data and AI-powered analytics with psychology could build a promotion to target said customer within minutes. This might involve offering a slight discount or a bundle that incorporates a premium offering with some medium-value accessories.
So, while legacy data can help build a picture, to move from general knowledge to actionable intelligence requires the right information, in the right place, in real time. This means being able to integrate new data and combine established patterns with up-to-date insights to determine the relevant actions.
For example, an airline knew that its customer relationships suffered when flights were delayed or cancelled. It wanted to identify and prioritize those customers most at risk due to these incidents and proactively offer personalized compensation to save the relationship and reduce customer defection on high-priority routes. To do that, it used a variety of customer, operations and financial variables in a machine-learning application to measure both satisfaction and predicted revenue for its more than 100 million customers every day. It ultimately resulted in an 800% increase in satisfaction and a 60% reduction in priority customer churn.
This was based on fast access to the right intelligence, combined with a system that worked, learned and adapted in real time. This means that businesses targeting new customer types or building new services to meet changing customer expectations can be just as effective as those B2C brands that have years of experience.
A constantly changing customer
Of course, every company wants to take that next step, whether a mature user of data trying to speed up their time to market, or a legacy player still trying to manage a complex transformation and get a better handle on their data landscape. What they all need to realize is that the customer is constantly changing, with evolving expectations. So, any understanding businesses have of the customer is also in an ongoing state of disruption. Past behavior is not a guarantee of future performance; it can provide a guide of what may happen, but it should not be taken as an absolute certainty.
To combat that, companies must get themselves in the best possible position, by having access to the right data, being able to generate insights from it and use AI tools to apply those learnings in real time, all informed by an awareness of what customers really want.
Using data effectively is a critical part of delivering an exemplary customer experience. Find out more here on how you could incorporate data insights into your customer experience efforts.
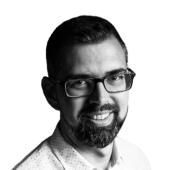
In my engagements, I leverage 20 years of experience on digital ecosystems, customer engagement and AI, IoT and advanced analytics. Keys to success include improving customer experiences, improving processes and organizations, defining winning KPI’s and unlocking customer insights. I am a member of the Business & Decision Europe Regional Board and Lead for Customer Engagement at Business & Decision Europe.