Big data is now critical to business success. By 2022, 90% of corporate strategies will explicitly mention information as a critical enterprise asset and analytics as an essential competency, according to Gartner. It estimates that by 2021 enterprises using a cohesive strategy incorporating data hubs, lakes and warehouses will support 30% more business use cases than their competitors. This will allow them to exploit new business opportunities better and more quickly, for example.
With the desire to aggregate disparate data sets fast, many enterprises are looking for a quick and reliable way to migrate their data into data lakes. Cloud-based data lakes are often preferred because of their greater agility, flexibility and scalability. Creating a data lake and migrating the data to it can be a complex undertaking that can need consultancy and an experienced partner. Data engineers are required, for example, to get data out of source systems and feed it into the data lakes. Data cleaning and enrichment are also important tasks in the ingest process.
Data lake vs. data warehouse
Data lakes and data warehouses are both data storage repositories that provide one single point of truth (SPOT). But there are key differentiators: data lakes generally store raw, unprocessed data in its native format, which can include unstructured, semi-structured and structured data, while data warehouses contain processed and aggregated data.
Data lakes give users easy access to different sources of data, making them ideal for data scientists and data analysis experts. The data lake is designed for storing data as-is, in its native format. This means new sources can be instantly added, and analysis can be done quickly. With data lakes, enterprises can run analytics without moving data to a separate analytics system. Data warehouses, on the other hand, are highly structured, and changes can be made to the structure, but it can be time consuming.
Data stored in data lakes, however, needs to be cataloged and indexed to make real sense of it. This is a manual process and takes time. If this issue isn’t addressed from the outset, an enterprise can quickly find itself with a useless data swamp.
Disorganized data can also cause major security issues as the surface area of the data lake is very large. Therefore, a data governance process and essential security policies must be put in place to maintain security of data assets in the data lake.
The big data challenge
According to Aberdeen Research, the average enterprise’s data volume is growing at a rate that exceeds 50% per year. This size of growth can increase complexity and create internal efficiency challenges, especially where enterprises are heavily dependent on data.
Aberdeen Research maintains that the agility and scalability provided by data lakes saw enterprises that successfully deployed data lakes outperform competitors by 9% in revenue growth. This is primarily due to the fact that they were able to easily exploit emerging analytics technologies such as machine learning over new data sources such as social media and click-streams. The report also found that data lakes enable enterprises to spot new opportunities in data faster, letting them make informed decisions quicker thereby boosting productivity.
Data lakes in the cloud
Data lakes lend themselves to cloud deployment because the cloud environment offers performance, faster deployment, scalability, reliability and access to a number of analytic engines – including those that analyze data from IoT devices.
Many cloud providers provide technology for building data lakes in the cloud, including AWS, Microsoft Azure, and Flexible Engine from Orange Business. While compliance and regulatory requirements are covered by the big vendors, the cloud isn’t free of operational obligations: if you have problems with data loads you will need an operator who understands the environment.
Loading and storing data in the cloud may be inexpensive, but if you want to get your data back onto your own systems or replicate it over availability zones, it can be expensive. Enterprises need to consider if there are any events that will necessitate doing this, and they could look to move to a hybrid cloud environment and want to take some data back in-house, for example.
Moving to in-cloud data lakes
Moving data to a data lake in the cloud is not something that happens overnight. It needs to be carefully thought out to control costs and any business disruption.
Enterprises need to seek out business cases that will benefit from data being moved to a cloud-based data lake in the first instance. Once these are successfully migrated, a strategy can be determined for the rest of the data. Integration needs to be built with the on-premises platform as well as in the cloud.
If an enterprise is looking at data lakes in multi-cloud environments, then the complexity issue grows with different vendors and technologies to contend with. Enterprises need to check, for example, they aren’t looking at vendor lock-in.
Making the cloud call
Moving your data from an on-premises cluster to a cloud-based platform is much easier than it used to be. As I mentioned previously, the big cloud vendors like AWS, Microsoft Azure all have cloud services that allow enterprises to build data lakes. But it is still a big challenge: data lakes primarily fail because the homework and pre-planning hasn’t been done thoroughly.
Data lakes need to be built in accordance with an enterprise’s specific needs today and going forward, otherwise they will end up as messy data swamps. The same applies to data lakes in the cloud. They require the right data storage infrastructure and data management architecture to be put in place to work effectively.
Enterprises should always draw up a business case for data lakes, be they on-premises or in the cloud. If an enterprise can’t pinpoint any pre-defined cases, then it needs to determine if a data lake is really the right route to go. This is where expert consultancy and experienced partners can provide the answers and the skillsets.
For more information on data lakes and how to generate more value from your data, download our brochure: Make your analytics more agile.
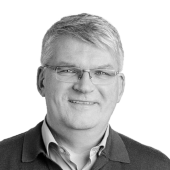
Ingo Steins is The unbelievable Machine Company's Deputy Director of Operations, heading up the applications division in Berlin. He joined *um two years ago as a Development Team Leader – coming with many years of experience in software and data development and in managing large teams. He now manages three large teams at *um. Ingo is an expert in business intelligence, data management and big data.