As businesses deploy digital infrastructures and look at ways to leverage AI and Generative AI (GenAI) in their day-to-day operations, often by relying on cloud services, a foundational layer that combines compute and network connectivity is critical. If there is no network, there is no cloud and no AI.
This puts the network front and center for enabling digital business.
AI and the network
So, as we’re in the midst of the AI boom, what does this mean for the network?
It’s important to point out that no one can predict the future, and, as with the Internet in the late 1990s, we are likely to make less-than-accurate predictions, especially as AI use cases are just starting to develop, and most companies are still at the beginning of their AI journeys.
It is easy to hear how AI might be used and what that might mean for the business, but ultimately, the use cases that will shape deployment are still being defined. That has significant implications for the network.
We already know that GenAI alone requires significant infrastructure to support it; one study found that 52% of GenAI investments are going to dedicated or public cloud infrastructure in the next 18 months.
Network’s twin AI requirements
From a network perspective and, again, based on early, weak signals, demand is likely to force change in two ways.
First, there are the supporting, data-hungry AI applications. GenAI foundational models require huge volumes of data to train them and, ultimately, for them to be used effectively. Handling that data requires flexible storage, which requires cloud computing and high-speed, agile connectivity.
When these models are deployed in applications, they also need low latency to respond as users expect. No consumer is going to wait for a chatbot that takes ten minutes to respond to a question; likewise, a quality-assurance AI visually inspecting manufacturer components would slow down production if it took too long to do its job.
Second, data is created outside of traditional data centers and clouds. Whether AI applications are embedded in edge devices or hosted in centralized environments, reliance on AI to function properly, a network with the capacity, resiliency, bandwidth and latency to handle data-in-motion is needed.
This is a step change for the network. In the cloud era, the direction of travel was mainly cloud (or data center) to site, with a small amount of data going from site to cloud. With AI, this is quite likely to change with data indistinctively traveling from cloud to site, site to cloud, site to site and cloud to cloud. This will put stress on network infrastructure that is more and more designed for cloud to site traffic patterns.
Transforming the network
Network infrastructures will need to be rethought to meet use-case needs and because the future is unknown. The principles that have guided the design, delivery and run for adopting network as a service are fundamentally transforming how customers procure and provision services to ensure that they can quickly and efficiently adapt with whatever AI strategy is deployed by the business.
So how do enterprises create this sort of AI-capable network, particularly when they are still building their own AI plans?
One Orange Business customer needed to get data from several data centers worldwide to its devices. Due to sovereignty rules at one specific location, they had to build a dedicated data center for that country. Because of this decentralized storage, the amount of data in motion increased, putting strain on existing networks that struggled to meet the customer’s need for speed, reliable connectivity and low latency. To get around this, the company built its own private network.
That company was one of the world’s most well-resourced, handling extremely high-value data. It won’t always be feasible for other companies to go to this extent.
Building a new network strategy
Enterprises should consider developing a network strategy that:
1. Plans for the future
Use cases determine AI deployment – it’s that simple. By defining their most likely use case(s), enterprises can assess their network requirements and transformation path. These use cases should reflect where the AI is hosted, how it is used and how decentralized the business and data are. They will also need to incorporate the levels of compute power deployed, connectivity speeds and an understanding of how data will move to contribute to a total cost of ownership.
2. Prioritizes privacy, security and regulatory compliance
Data storage is increasingly decentralized, which means more data is in transit, potentially making the data more complex to secure. Therefore, there is a greater need to holistically secure the elements of the infrastructure that the data traverses without compromising the use of AI.
In addition to the physical security of data, companies of all sizes must be aware of the implications, including from a regulatory standpoint, of having their data in multiple evolving environments.
As such, we believe that the networks connecting data to users must contribute to and allow enterprises to remain compliant, while having the flexibility to meet the evolving needs of data in transit.
3. Enables good governance
Linked to security, privacy and regulation is AI governance. Poor governance leads to errors, breaches and exposure; networks can help enterprises avoid that. Alongside knowing who manages data and where it comes from, you also need to have clear visibility of your infrastructure, including the network. If you can trust your network and know that it’s secure and doesn’t compromise data privacy, then you are much closer to trusting the data feeding into your AI.
4. Handles congestion, traffic and disruption
As we’ve already discovered, the traffic your networks handle will grow, or at least the patterns and needs will change. Add more cars onto a road, and eventually you’ll get congestion if you don’t manage those roads appropriately. It’s the same with networks: you'll run into speed and latency issues if you don’t manage your network deployments.
The network must also handle the reality of the world today. Networks can be affected and targeted by natural disasters and geo-political conflict, which can lead to service degradation and challenges, not only in the cloud but also on the edge (at your sites). This means network strategies need contingencies that can meet service level agreements no matter what happens – from the user to the data.
An on-demand network fit for AI
We cannot wait to see what AI will bring and how businesses will use it to enrich their business models; but, like all business-model transformations, AI will have a significant impact on how businesses are run and how they consume and utilize digital infrastructure, including secured networks.
At Orange Business, we are transforming our core product offerings into a future-proof, secure network value proposition that is delivered as a service and that will adapt, flex and scale to accommodate our customers’ needs, whatever the use case, to support enterprises in realizing the full potential of AI.
AI is transforming the network. What does it mean for security, data and more? Find out in our new guide: Your route to AI success.
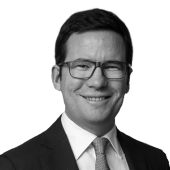
Pierre-Marie Binvel is Director of Connectivity Product Marketing at Orange Business, managing all connectivity and SASE product development and marketing activities for B2B markets. In this capacity, Pierre-Marie leads the creation of Evolution Platform, the Orange Business market-leading B2B multi-service platform.